Falls are often referred to as geriatric giants. They represent a vast public health problem for the elderly population. Most falls do not result in any injuries, and could be thought of as “harmless giants”. However, others are indeed dreadful and dangerous creatures – either because they result in severe injuries or in a detrimental change of a person’s mental state, often referred to as fear of falling. If we would be better at predicting who is likely to fall in the future, we might be better at identifying an effective strategy to prevent falls. Yet, as Niels Bohr once said, “prediction is very difficult, especially about the future”.
Modern machine learning techniques can help us to make better predictions. These learning machines can use two different kinds of “fuel”; i.e. prior knowledge and empirical training examples. FRAT-up is a predictive tool for falls which we have developed exclusively using knowledge from scientific medical literature (i.e. prior knowledge). As part of the current study, we have explored the use of data from a large dataset as alternative “fuel” for our learning machine. We exploited the extensive InCHIANTI dataset about mobility and falls in the elderly and compared our results with simple fall risk indicators (e.g. falls history, gait speed, Short Physical Performance Battery). These indicators are commonly used in clinical practice when stratifying older people according to their fall risk. Our analysis led to the development of a predictive model for falls with a predictive ability that is very similar to the one exhibited by FRAT-up. Both approaches clearly outperformed the common simpler fall risk indicators.
In conclusion, our results confirm the validity of FRAT-up and its literature-based approach. Furthermore, our analyses have highlighted that the complexity of the causes underlying falls cannot be oversimplified in predictive tools, without affecting their predictive ability. The multifactorial nature of falls has to be faced and managed, in order to win the battle against this geriatric giant.
Figure: ROC curves of the data-driven model (Lasso) and the other risk indicators (number of previous falls, gait speed, Short Physical Performance Battery, and FRAT-up) when predicting for single (left panel) and multiple (right panel) falls. doi:10.1371/journal.pone.0146247.g002
Publication
Palumbo P, Palmerini L, Bandinelli S, Chiari L (2015) Fall Risk Assessment Tools for Elderly Living in the Community: Can We Do Better? PLoS ONE 10(12): e0146247. doi:10.1371/journal.pone.0146247
http://journals.plos.org/plosone/article?id=10.1371/journal.pone.0146247
About the Author
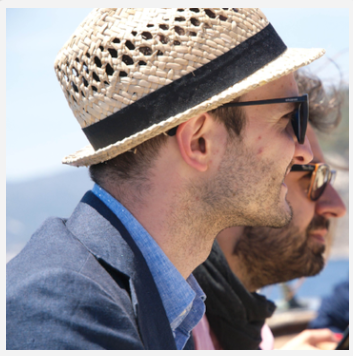
Pierpaolo Palumbo
Biomedical Data Analysis
Pierpaolo Palumbo received his MSc in Medical Engineering in 2011 at the University of Rome Tor Vergata and his PhD in Bioengineering in 2015 at the University of Bologna. He works on biomedical data analysis, addressing topics related to ageing and prevention.
Copyright
© 2018 by the author. Except as otherwise noted, the ISPGR blog, including its text and figures, is licensed under a Creative Commons Attribution-ShareAlike 4.0 International License. To view a copy of this license, visit https://creativecommons.org/licenses/by-sa/4.0/legalcode.
ISPGR blog (ISSN 2561-4703)
Are you interested in writing a blog post for the ISPGR website? If so, please email the ISGPR Secretariat with the following information:
- First and Last Name
- Institution/Affiliation
- Paper you will be referencing