Wearable sensors have become an important research tool because they can be used to monitor physical movement remotely. This makes it possible to estimate how much energy a person expends when they perform their daily activities and can help in the management of certain chronic diseases (e.g., diabetes and obesity) which are often managed through increasing regular physical activity levels. In this scenario, the accuracy of an activity classification algorithm becomes pivotal because it directly influences the estimate of a person’s energy expenditure. The current study aimed to compare the accuracy of an activity classification algorithm that is trained on younger adults and evaluated with data collected from older adults and vice versa.
Thirty seven older adults (83.9 ± 3.4 years) and twenty younger adults (21.9 ± 1.65 years) were asked to perform several activities of daily living under semi-supervised free-living conditions whilst carrying a smartphone in their pants pocket. The measurements from the smartphone’s sensors (accelerometer, gyroscope, and barometric air pressure sensor) were recorded whilst participants walked (both on level ground as well as upstairs and downstairs), remained stationary (i.e., standing, siting and lying) or transitioned between different sedentary positions. The accuracy of the activity classification algorithm developed was lower when the algorithm was trained on the data collected from the younger cohort and tested on the data collected from the older cohort, particularly when trying to identify periods of stair ascent and descent. When the mean value of the differential pressure feature (see Figure 1.) across the younger cohort was compared to the mean value across the older cohort, the magnitude of the value was significantly smaller (p< 0.001) which suggests that the older adults ascended and descended the staircases at a slower rate compared to the younger adults.
The study shows that the accuracy of an activity classification algorithm is dependent on the characteristics of the cohort on which it is trained. This is an important consideration to keep in mind, especially when using the same algorithm across different age groups, or in people with different physical characteristics due to certain clinical conditions (e.g., Multiple sclerosis, Parkinson’s disease). Future algorithm development for activity classifications should focus on fine-tuning algorithms based on the populations in which it will be used.
Publication
Del Rosario, M., Wang, K., Wang, J., Liu, Y., Brodie, M., Delbaere, K., Lovell, N., Lord, S. and Redmond, S. (2014). A comparison of activity classification in younger and older cohorts using a smartphone. Physiol. Meas., 35(11), pp.2269-2286.(http://iopscience.iop.org/article/10.1088/0967-3334/35/11/2269)
About the Author
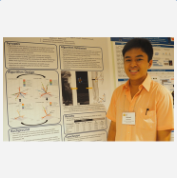
Michael Benjamin Del Rosario
Graduate School of Biomedical Engineering, The University of New South Wales
Michael Benjamin Del Rosario is a PhD Candidate at the Graduate School of Biomedical Engineering, The University of New South Wales, Sydney, Australia.
Michael’s research aims to develop algorithms that can identify physical activities using measurements from wearable sensors, and incorporate them into telehealth solutions that can be used to remotely monitor an individual’s physical activity over extended periods of time. He is currently investigating the utility of a smartphone-based telehealth application as an adjunct to an outpatient cardiac rehabilitation program during the final phase of his PhD programme.
Copyright
© 2018 by the author. Except as otherwise noted, the ISPGR blog, including its text and figures, is licensed under a Creative Commons Attribution-ShareAlike 4.0 International License. To view a copy of this license, visit https://creativecommons.org/licenses/by-sa/4.0/legalcode.
ISPGR blog (ISSN 2561-4703)
Are you interested in writing a blog post for the ISPGR website? If so, please email the ISGPR Secretariat with the following information:
- First and Last Name
- Institution/Affiliation
- Paper you will be referencing